Adapting LLM & NLP Models to Domain-Specific Data 10x Faster with Better Data
When building high-performing LLM & NLP systems, most of our time is spent debugging models and iterating over individual issues that lead us to fixing our datasets in an ad-hoc, manual way.
Using data-centric algorithms on signals from models can provide us with better insights and patterns about cases for which our models are likely to fail during training as well as in production.
This session shares examples of these best practices in action using the integration between the Galileo and John Snow Labs platforms, showing how to quickly identify & fix issues during both training and inference.
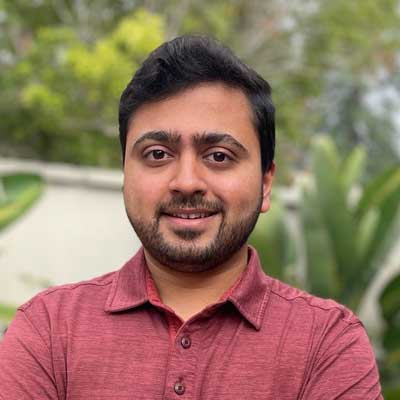
Yash Sheth
Co-Founder at Galileo
Yash is the co-founder and COO at Galileo — an evaluation, experimentation, and observability platform for building trustworthy LLM applications.
Prior to Galileo, Yash led the speech platform team at Google, where his team worked on building and scaling to thousands of speech recognition models to serve 20+ Google products. Yash was an early engineer on Google Assistant, built Google’s Cloud Speech API and grew it from 0 to thousands of organizations.
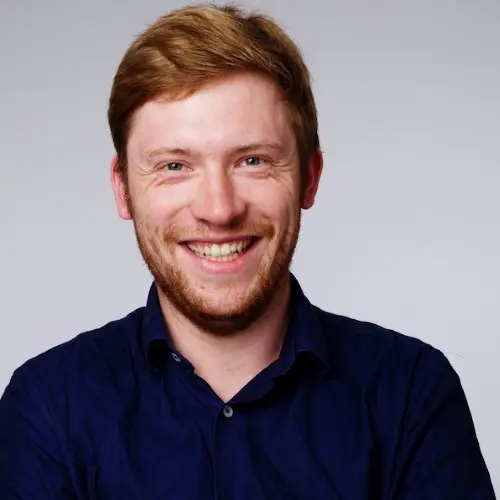
Franz Keller
Platform Lead at Galileo
Coming soon
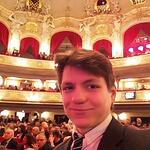
Christian Kasim Loan
Lead Data Scientist at John Snow Labs
Christian Kasim Loan is a Lead Data Scientist and Scala expert at John Snow Labs and a Computer Scientist with over a decade of experience in software and worked on various projects in Big Data, Data Science and Blockchain using modern technologies such as Kubernetes, Docker, Spark, Kafka, Hadoop, Ethereum, and overr 20 programming languages to create modern cloud-agnostic AI solutions, decentralized applications, and analytical dashboards.
He has deep knowledge of Time-Series Graphs from his previous research in scalable and accurate traffic flow prediction and working on various Spatio-Temporal problems embedded in streaming graphs at a Daimler lab.
Before his graph research, he worked on scalable meta machine learning, visual emotion extraction, and chatbots for various use cases at the Distributed Artificial Intelligence lab (DAI) in Berlin.
His most recent work includes the NLU library, which democratizes 10000+ state-of-the-art NLP models in 200+ languages in just 1 line of code for dozens of domains, with built-in visualizations and all scalable natively in Spark Clusters by its underlying Spark NLP distribution engine.