Applying Healthcare-Specific LLMs to Build Oncology Patient Timelines and Recommend Clinical Guidelines
The emergence of precision oncology necessitates a comprehensive understanding of how genetic, epigenetic, and other factors influence tumor behavior and response to treatment regimens. This understanding is crucial for translating research findings into patient-specific therapies.
In this talk, I will cover two case studies. First, I will discuss how applying healthcare-specific Large Language Models (LLMs) to Electronic Health Records (EHRs) presents a promising approach to constructing detailed oncology patient timelines. This task involves extracting and synthesizing chemotherapy treatment data from diverse clinical notes, including those from primary care providers, oncologists, discharge summaries, emergency departments, pathology, and radiology reports.
Second, I will explore how John Snow Labs’ healthcare-specific Large Language Model (LLM) offers a transformative approach to matching patients with the National Comprehensive Cancer Network (NCCN) clinical guidelines. By analyzing comprehensive patient data, including genetic, epigenetic, and phenotypic information, the LLM accurately aligns individual patient profiles with the most relevant clinical guidelines. This innovation enhances precision in oncology care by ensuring that each patient receives tailored treatment recommendations based on the latest NCCN guidelines.
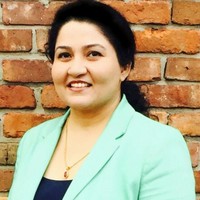
Vishakha Sharma
Senior Principal Data Scientist at Roche