Automated Bias, Robustness, and Data Quality Testing for NLP Models
As the use of machine learning (ML) algorithms in private and public sectors continues to expand, there are growing concerns about robustness, fairness, and bias in the ways in which machine learning models are developed and used in simple and complex decision-making processes.
In this talk, Veysel will present his team’s latest research and efforts towards mitigating the brittleness and vulnerabilities of ML models in real world settings, particularly in healthcare domain wherein biased algorithms can have life threatening impacts.
During his talk, Veysel will explain how to measure robustness and bias of medical NLP models without using any labelled datasets and then also offer out-of-the-box solutions regarding how to fix them with a few lines of code. He will also share some insights on how to detect labelling errors before training the models and incorporate the fixing strategies in a close coordination with data science and annotation teams.
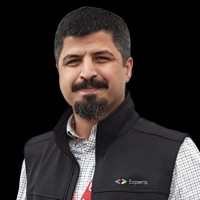
Veysel Kocaman
Head of Data Science at John Snow Labs
Veysel is a Lead Data Scientist and ML Engineer at John Snow Labs, improving the Spark NLP for the Healthcare library and delivering hands-on projects in Healthcare and Life Science.
He is a seasoned data scientist with a strong background in every aspect of data science including machine learning, artificial intelligence, and big data with over ten years of experience. He’s also pursuing his Ph.D. in ML at Leiden University, Netherlands, and delivers graduate-level lectures in ML and Distributed Data Processing.
Veysel has broad consulting experience in Statistics, Data Science, Software Architecture, DevOps, Machine Learning, and AI to several start-ups, boot camps, and companies around the globe. He also speaks at Data Science & AI events, conferences and workshops, and has delivered more than a hundred talks at international as well as national conferences and meetups.