Automated de-identification of medical documents & images
One kind of noisy data that healthcare data scientists deal with is scanned documents and images: from PDF attachments of lab results, referrals, or genetic testing to DICOM files with medical imaging.
These files are challenging to de-identify because personal health information (PHI) can appear anywhere in free text – so cannot be removed with rules or regular expressions – or “burned” into images so that it’s not even available as digital text, to begin with.
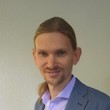
AMykola Melnyk is a senior Scala, Python, and Spark software engineer with 15 years of industry experience.
He has led teams and projects building machine learning and big data solutions in a variety of industries - and is currently the lead developer of the Spark OCR library at John Snow Labs.
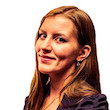
Alina is a senior data scientist at John Snow Labs, where she is working as a part of the Core team. Her recent work was focus on creating models and pipelines to process healthcare documents for the purposes of Named-entity recognition and de-identification. John Snow Labs is a healthcare AI company, accelerating progress in data science by providing state-of-the-art models, data, and platforms.
Before joining John Snow Labs she was working for more than 5 years as a consultant in the area of NLP, Image recognition, and Predictive modeling and completed her Ph.D. studies in Apply Mathematics. In her free time, she enjoys hiking and watching indie movies.