Capabilities of LLMs and Few-Shot Learning: A study on Cardiac MRI Data
Clinical decision making is often based on analysis of retrospective patient cohorts to enable and guide treatment decisions. In general, there are large volumes of data within hospitals within Electronic Patient Record systems as unstructured data and some of the challenges in extracting relevant information involves manual resource from the limited number of expert annotators with sufficient domain knowledge.
In this study, we explored the use of Large Language Models and prompting-based techniques to extract information from CMR reports that contain patient information and multiple measurements, which otherwise would require manual extraction and transcription to databases without errors.
We have also evuated on training customised models in a few-shot setting when minimal annotated data is available and computational resources such as GPUs are not. Our study evaluates the adaptability and performance of LLMs on our hospital data can provide useful insights for applications in other real-world settings.
About the speakers
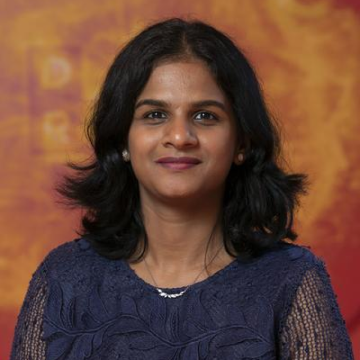
Pavithra Rajendran
NLP Technical Lead and Senior Data Scientist at Great Ormond Street Hospital NHS Trust
Pavi joined the GOSH DRIVE team in July 2022 and has around 7 years of academic and commercial experience as an NLP (Natural Language Processing) specialist. She is currently leading several NLP projects, in particular, focussing on developing and deploying modularised and reusable NLP pipelines for extracting relevant structured information from unstructured text data. Previously, worked at KPMG UK as an NLP data scientist and has experience using both traditional and deep learning-based NLP techniques for various client projects in both public and private sectors, from Proof-of-Concept to Production (Healthcare, Oil and Gas, Travel, Finance etc.) and, translating academic knowledge/research for commercial settings as well as latest commercial technologies by identifying the gaps and presenting with appropriate solutions for the business problems. She holds a Masters in Advanced Computing (Machine Learning, Data Mining and High Performance Computing) and a PhD in Computer Science (Natural Language Processing).
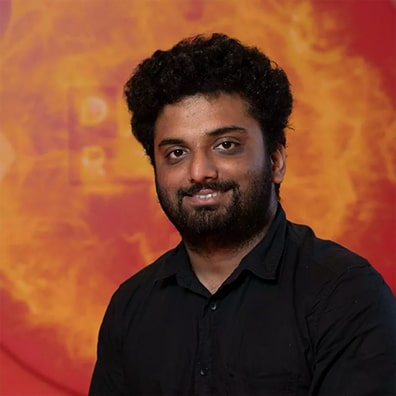
Sebin Sabu
NLP Data Engineer at Great Ormond Street Hospital NHS Trust