Clustering Patients Complaints
This talk delves into the innovative application of clustering algorithms to analyze patient complaints in the Emergency Room (ER). The primary objective is to enhance the understanding of patient needs and optimize ER operations. We start by outlining the significance of effectively managing patient complaints, emphasizing its impact on healthcare quality and patient satisfaction.
The methodology section describes the data collection process, including the categorization of complaints into various domains such as wait times, medical care, staff behavior, and facility amenities. We then introduce the clustering techniques used, such as K-means and hierarchical clustering, and explain how these methods help in identifying patterns and trends in the complaints.
The results section presents a comprehensive analysis, highlighting key clusters of complaints and their implications for ER management. This includes insights into the most frequent and critical complaints, enabling targeted improvements in ER services. The talk concludes by discussing the broader implications of this study for healthcare policy and practice. We emphasize how data-driven approaches like clustering can lead to more patient-centered care and efficient healthcare delivery.
The potential for scalability and application in other healthcare settings is also explored, along with future directions for research in this field. This abstract summarizes the key points of the talk, including the motivation, methodology, results, and implications of clustering patient complaints in an ER setting.
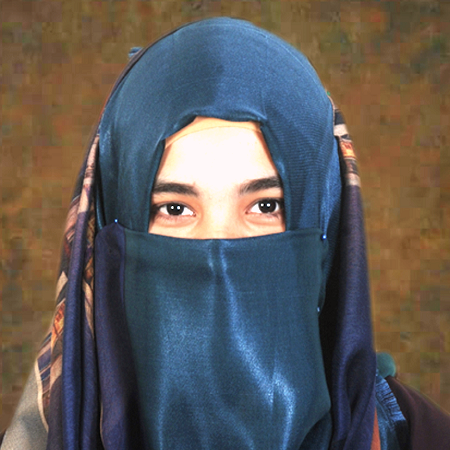
Zarmeen Nasim
Senior Instructor at Aga Khan University
Dr. Zarmeen completed her doctoral studies in Computer Science from IBA in 2022. She has been a part of the Artificial Intelligence (AI) lab at the Institute of Business Administration (IBA), Karachi. ‚ÄãDuring her Ph.D., she worked on several research projects that primarily focused on using natural language processing techniques for solving real-world problems. Her research interests include text analytics, data mining, social network analysis, and machine learning. In her current role at AKU, she aims to address health-related problems using data science and machine learning methods.