Efficiency is the New Precision
The global data sphere consisting of machine data and human data is growing exponentially reaching into the Zettabyte scale. Compared to this, the processing power of computers has been stagnating for many years. Artificial Intelligence – a most recent variant of Machine Learning – circumvents the necessity of understanding a system when modelling it; but this convenience comes with extremely high energy consumption.
The complexity of language makes statistical Natural Language Understanding (NLU) models particularly energy-hungry. As the biggest part of the Zettabyte data sphere consists of human data, like text or social networks, we are facing 4 major impediments: 1. Findability of Information – when the truth is hard to find, fake news rule 2. Von Neumann Gap – when processors cannot process faster, then we need more of them (energy) 3. Stuck in the Average – when statistical models generate a bias toward the majority, innovation has a hard time 4. Privacy – if user profiles are created “passively” on the server-side instead of “actively” on the client-side, we lose control.
The current approach to overcome these limitations is to use ever-growing data sets on increasingly large numbers of processing nodes for training. AI algorithms should rather be optimized for efficiency instead of precision, in which case statistical modelling is a brute force approach should be disqualified for language applications. When replacing statistical modelling, Mengenlehre (set theory) seems to be a much better choice as it allows the direct processing of words instead of their occurrence counts, which is exactly what the human brain does with language – using only 7 Watts!
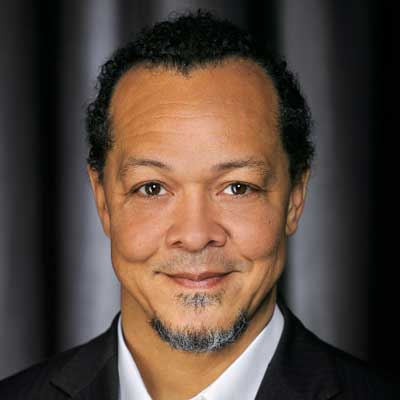
Francisco De Sousa Webber
CEO, Founder at Cortical.io
Francisco Webber, CEO and Co-founder of Cortical.io, is a visionary and seasoned executive leader in business management, IT, and R&D in AI & search engine technologies.
His medical background in genetics combined with over two decades of experience with search engine technologies, documentation systems and AI led him to formulate his theory of Semantic Folding, which models how the brain processes language data.
In 2011, he co-founded Cortical.io to apply the computational principles of the human brain to large scale text data. This novel way of machine learning allows us to solve real-world use cases in a highly efficient manner: Semantic Supercomputing.