EHR-Safe: Generating High-Fidelity and Privacy-Preserving Synthetic Electronic Health Records
Privacy concerns often arise as the key bottleneck for the sharing of data between consumers and data holders, particularly for sensitive data such as Electronic Health Records (EHR). This impedes the application of data analytics and ML-based innovations with tremendous potential. One promising approach to avoid such privacy concerns is to instead use synthetic data. We propose a novel generative modeling framework, EHR-Safe, for generating highly realistic and privacy-preserving synthetic EHR data.
EHR-Safe is based on a two-stage model that consists of sequential encoder-decoder networks and generative adversarial networks. Our innovations focus on the key challenging aspects of real-world EHR data: the data are heterogeneous, consisting of numerical and categorical features with distinct characteristics; they contain time-varying features with highly-varying sequence lengths; and the features are often highly sparse. Under numerous evaluations, we demonstrate that the fidelity of EHR-Safe is very high, i.e. it has almost-identical properties with real data while yielding almost-ideal performance in practical privacy metrics.
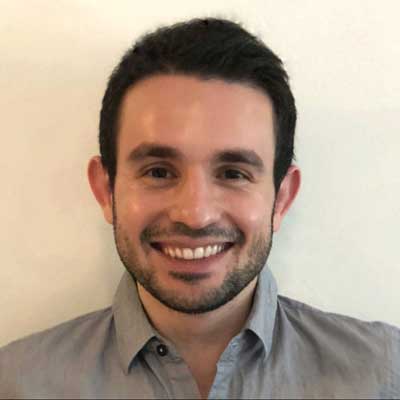
Sercan Arik
Research Scientist at Google
Sercan Arik is currently working as a Staff Research Scientist and Manager at Google Cloud AI Research. His current work is motivated by the mission of democratizing AI and bringing it to the most impactful use cases, from Healthcare, Finance, Technology, Retail, Media, Manufacturing, and many other industries. He focuses on how to make AI more high-performance for the most-demanded data types, interpretable, trustable, data-efficient, robust and reliable. He led research projects that were launched as major Google Cloud products and yielded significant business impact. Before joining Google, he was a Research Scientist at Baidu Silicon Valley AI Lab.
At Baidu, he has focused on deep learning research, particularly for applications in human-technology interfaces. He co-developed state-of-the-art speech synthesis, keyword spotting, voice cloning, and neural architecture search systems. He completed my PhD degree in Electrical Engineering at Stanford University. He has co-authored more than 50 journal and conference publications.
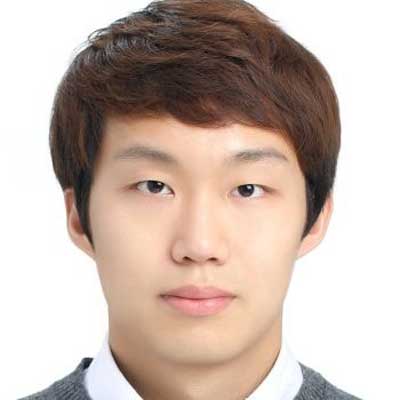
Jinsung Yoon
Research Scientist at Google
Jinsung Yoon is a senior research scientist at Google Cloud AI. He is currently working on diverse machine learning research topics such as generative models, self- and semi-supervised learning, model interpretation, data imputation, anomaly detection, and synthetic data generation. He received his Ph.D. and M.S. in Electrical and Computer Engineering Department at UCLA, and B.S. in Electrical and Computer Engineering at Seoul National University (SNU).
In 2021, he was selected as the innovator under 35 in South Korea from MIT Technology Review.