Extracting events and their dates from clinical text to generate real world evidence
Information captured in clinical text in the course of medical care is a rich potential source of research data. To be usable for research analyses such as comparative effectiveness studies, clinical events and characteristics must first be extracted in a structured form.
Extracting information from clinical text is a challenging problem for NLP algorithms because the text is inherently longitudinal, occurring over many notes in a sequence of visits. Accurately extracting the date of an event–for example a diagnosis, receipt of a drug, or a surgery–can be as important as extracting the event itself.
In this talk, I’ll present a deep learning architecture we’ve developed at Flatiron Health for extracting events and their dates from longitudinal clinical text. The architecture first encodes sentences potentially related to the event of interest from each note, then integrates across the patient chart using a novel time-aware aggregation layer. I’ll present results of using this architecture for extracting advanced diagnosis of non-small cell lung cancer, and discuss applications to other clinical events.
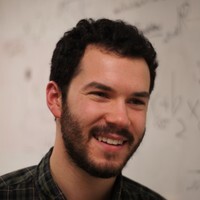
Alex Rich
Staff Data Science Manager at Flatiron Health
Alex is a Data Science Manager at Flatiron Health, where he works to develop NLP algorithms that can be used to generate high-quality real world datasets for cancer research. Along with his NLP work, he has also collaborated with biostatisticians to research techniques to account for ML-extracted variable uncertainty in downstream statistical analysis, and with healthcare practices to build point-of-care ML-based risk stratification tools.
Prior to Flatiron, Alex completed a PhD in cognitive psychology at New York University, where he researched biases in human learning and decision making and their relation to biases that can develop in machine learning systems.
When
Sessions: April 5th – 6th 2022
Trainings: April 12th – 15th 2022