Generative adversarial networks (GANs) and Bayesian analysis for incorporating expert opinions into advanced credit risk models
Credit risk Internal Ratings-Based (IRB) models are advanced methodologies used by banks to
calculate the minimum amount of capital they need to hold to cover potential losses from credit risk, as per regulatory frameworks such as the Basel II and Basel III accords. Under the Advanced IRB (A-
IRB) Approach, banks have greater flexibility and can estimate all the risk components themselves, such as Probability of Default (PD) and Loss Given Default (LGD). Whilst the A-IRB formula uses an asymptotic risk weight function to “stress” PD, it requires that the LGD in a downturn should be incorporated directly into the formula. This is a challenging task as downturn event data is unavailable unless the downturn has already occurred in the economy. Similar challenges also occur in the development of stress testing models which use unforseen macro-economic shocks and scenarios to stress the bank’s loss estimates. Therefore, alternative ways of modelling are required to make predictions in the absence of observed modelling data.
One of the effective ways is “Bayesian analysis”, where a Bayesian model is fit to make predictions for an economic downturn or an unforseen event, without the availability of the underlying empirical modelling data, by incorporating “expert” opinions into the model. The other effective way
is to using “Generative Adversarial Networks (GANs)” to simulate realistic financial losses via correlation matrices conditioned on the “expert” opinions of an economic downturn.
The talk begins with a background of the advanced IRB credit risk models followed by an overview of the modelling methodology covering the different challenges of developing models for economic
stress scenarios or downturn events. This is followed by a discussion on the underlying modelling problem case study and the rationale behind using alternative approaches – Bayesian analysis and GANs to solve that problem. The talk then moves on to discussing the “Bayesian analysis” solution covering key details and challenges encountered during the estimation process. It provides the technical use cases of making inferences using the Markov Chain Monte Carlo simulation techniques.
The talk then discusses an alternative methodology which would use GANs to generate realistic correlation matrices for a downturn event. The talk concludes by providing tips on explaining innovative modelling approaches to internal / external stakeholders who are usually familiar and comfortable with the traditional approaches, to ensure buy-in.
About the speaker
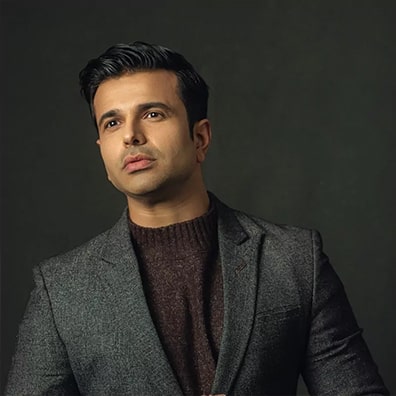
Varun Nakra
VP, Credit Risk at Deutsche Bank