Large Language Models for Biomedical Knowledge Graph Construction: Information Extraction from EMR Notes
The automatic construction of knowledge graphs (KGs) is an important research area in medicine, with far-reaching applications spanning drug discovery and clinical trial design. These applications hinge on the accurate identification of interactions among medical and biological entities. In this study, we propose an end-to-end machine learning solution based on large language models (LLMs) that utilize electronic medical record notes to construct KGs.
The entities used in the KG construction process are diseases, factors, treatments, as well as manifestations that coexist with the patient while experiencing the disease. Given the critical need for high-quality performance in medical applications, we embark on a comprehensive assessment of 12 LLMs of various architectures, evaluating their performance and safety attributes. To gauge the quantitative efficacy of our approach by assessing both precision and recall, we manually annotate a dataset provided by the Macula and Retina Institute. We also assess the qualitative performance of LLMs, such as the ability to generate structured outputs or the tendency to hallucinate.
The results illustrate that in contrast to encoder-only and encoder-decoder, decoder-only LLMs require further investigation. Additionally, we provide guided prompt design to utilize such LLMs. The application of the proposed methodology is demonstrated on age-related macular degeneration.
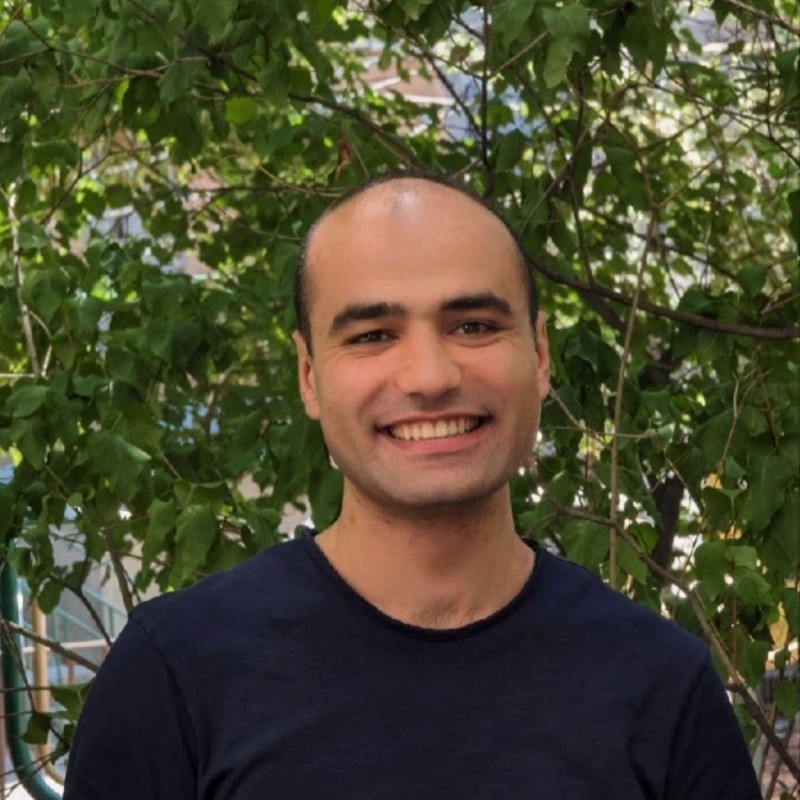
Davit Shahnazaryan
Head of Research at Amaros AI; Lecturer at Yerevan State University
Davit leads the research and machine learning at Amaros, where the focus is on accelerating clinical trials for chronic eye diseases through the utilization of machine learning for designing eligibility criteria, establishing external control arms, and enhancing patient recruitment. This technology is currently employed by 150 clinics, resulting in a recruitment acceleration of 20x-30x and a 5-10x expansion of the participant pool.
Davit oversees all aspects of technology, including both machine learning research and engineering. Simultaneously, he lecturing a course on deep learning and classical machine learning approaches to healthcare and drug discovery problems at Yerevan State University and the American University of Armenia.
Prior to his role at Amaros, Davit held research positions at KAUST and Wolfram Research. His academic background is in theoretical mathematics, specifically in logic, abstract algebra, and model theory.
When
Sessions: April 2nd – 3rd 2024
Trainings: April 15th – 19th 2024