Large Language Models Improve The Readability of Radiology Reports
With the increasing complexity of imaging modalities and the trend toward higher-level structured reporting, radiology reports have become equally as complex and lengthier by default, becoming nearly incomprehensible to the average person (patient). Additionally, there are ongoing efforts to produce patient-consumable radiology reporting. However, improving radiologist report structure and content is a necessary first step to consistently structured reports for clinicians’ ease of reading. More concise reports with predictable fields in anatomically oriented top-down logical order more effectively convey meaning. We listed and removed well-known unnecessary words (noise) common in radiologist reports that do not help convey meaning, resulting in an easier-to-read objective report (signal). This was followed by prompting the higher signal-to-noise ratio (SNR) phrases to read in plain English.
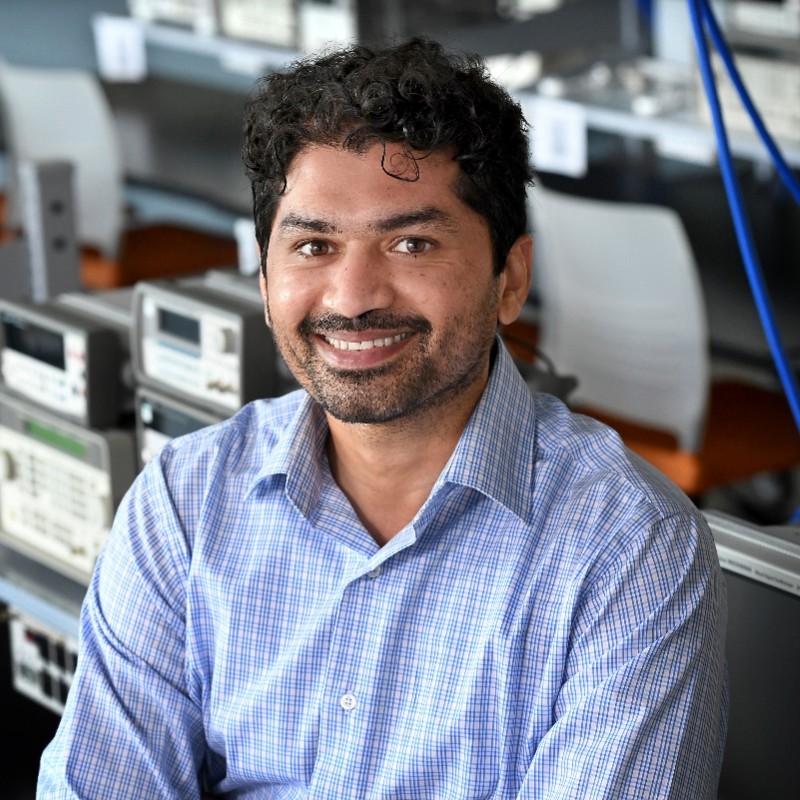
Ghualm Rasool
Assistant Professor at Moffitt Cancer Center
Ghulam Rasool is an Assistant Member in the Department of Machine Learning at the H. Lee Moffitt Cancer Center & Research Institute, Tampa, FL. He received a BS in Mechanical Engineering from the National University of Sciences and Technology (NUST), Pakistan, in 2000, an M.S. in Computer Engineering from the Center for Advanced Studies in Engineering (CASE), Pakistan, in 2010, and a Ph.D. in Systems Engineering from the University of Arkansas at Little Rock in 2014. He was a postdoctoral fellow with the Rehabilitation Institute of Chicago and Northwestern University from 2014 to 2016. Before joining Moffitt, he was an Assistant Professor at the Department of Electrical and Computer Engineering at Rowan University. His current research focuses on building trustworthy multimodal machine learning and artificial intelligence models for cancer diagnosis, treatment planning, and risk assessment. His research efforts are funded by the National Science Foundation (NSF), the National Institute of Health (NIH), and the Moffitt Cancer Center.