Learning to Summarize Visits from Doctor-Patient Conversations
Following each patient visit, physicians must draft a detailed clinical summary called a SOAP note. Moreover, with electronic health records, these notes must be digitized. Despite the benefits of this documentation, their creation remains an onerous process, contributing to increasing physician burnout.
In this paper, we present the first study to evaluate complete pipelines to train summarization models to generate these notes from conversations between physicians and patients.
We benefit from a dataset that, along with transcripts and paired SOAP notes, consists of annotations marking noteworthy utterances that support each summary sentence. We decompose the problem into extractive and abstractive subtasks, exploring a spectrum of approaches according to how much they demand from each component. We observe that the performance improves as we shift the burden to the extractive subtask.
Our best performing method first (i) extracts noteworthy utterances via multi-label classification, assigning each to summary section(s); (ii) clusters noteworthy utterances on a per-section basis; and (iii) generates the summary sentences by conditioning on the corresponding cluster and the subsection of the SOAP sentence to be generated.
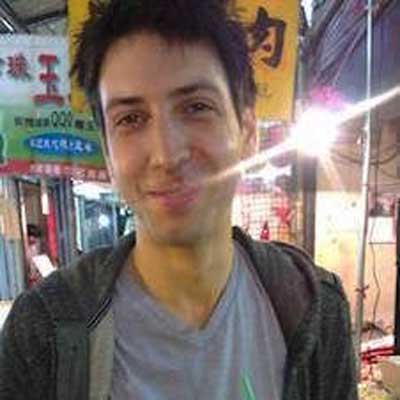
Zachary Lipton
Assistant Professor of Operations Research & Machine Learning at CMU
Zachary Chase Lipton is the BP Junior Chair Assistant Professor of Operations Research and Machine Learning at Carnegie Mellon University and a Visiting Scientist at Amazon AI.
His research spans core machine learning methods and their social impact and addresses diverse application areas, including clinical medicine and natural language processing. Current research focuses include robustness under distribution shift, breast cancer screening, the effective and equitable allocation of organs, and the intersection of causal thinking and messy high-dimensional data.
He is the founder of the Approximately Correct blog (approximatelycorrect.com) and a co-author of Dive Into Deep Learning, an interactive open-source book drafted entirely through Jupyter notebooks. Find on Twitter (@zacharylipton) or GitHub (@zackchase).