Linguistically informed NLP for healthcare experience data
Investigation of attention mechanisms of BERT and other language models – neural and otherwise – have found that these models are often, to one degree or another, implicitly learning details of syntactic structure and other linguistic features in the performance of various natural language processing tasks (Clark et al. 2019, Goldberg 2019, Shi et al. 2016, inter alia).
While this might be heartening news to linguists, it raises the questions of whether these frameworks might be partially reinventing the wheel with respect to the scientific knowledge of language, and also of what further linguistic structures and phenomena might be exploited to supplement the statistical models.
In this talk, I discuss various ways of leveraging linguistic structure to improve and expand NLP performance, particularly in a pipeline architecture, with a focus on experience data in the healthcare domain.
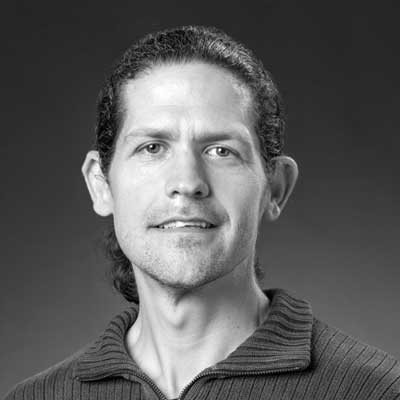
Zach Childers
Data Science Lead at Press Ganey
Zach Childers has worked for nearly five years in Natural Language Processing in health care, at NarrativeDx and continuing through their acquisition by Press Ganey.
Prior to that, he was trained as a linguist, working primarily on formal syntax, lexical semantics, and sentiment in English.
He is being awarded a forthcoming patent by the US Patent and Trademark Office covering technologies for valid dependency parsing and sentiment domain identification.
Recent publications include “Using AI to Understand the Patient Voice During the Covid-19 Pandemic” in the New England Journal of Medicine – Catalyst.