Machine Reading for Precision Medicine
The advent of big data promises to revolutionize medicine by making it more personalized and effective, but big data also presents a grand challenge of information overload.
For example, tumor sequencing has become routine in cancer treatment, yet interpreting the genomic data requires painstakingly curating knowledge from a vast biomedical literature, which grows by thousands of papers every day. Electronic medical records contain high-definition patient information for speeding up clinical trial recruitment and drug development, but curating such real-world evidence from clinical notes can take hours for a single patient.
Natural language processing (NLP) can play a key role in interpreting big data for precision medicine. In particular, machine reading can help unlock knowledge from the text by substantially improving curation efficiency. However, standard supervised methods require labeled examples, which are expensive and time-consuming to produce at scale.
In this talk, I’ll present Project Hanover, where we overcome the annotation bottleneck by combining deep learning with probabilistic logic, by exploiting self-supervision from readily available resources such as ontologies and databases, and by leveraging domain-specific pretraining on the unlabeled text.
This enables us to extract knowledge from tens of millions of publications, structure real-world data for millions of cancer patients, and apply the extracted knowledge and real-world evidence to supporting precision oncology.
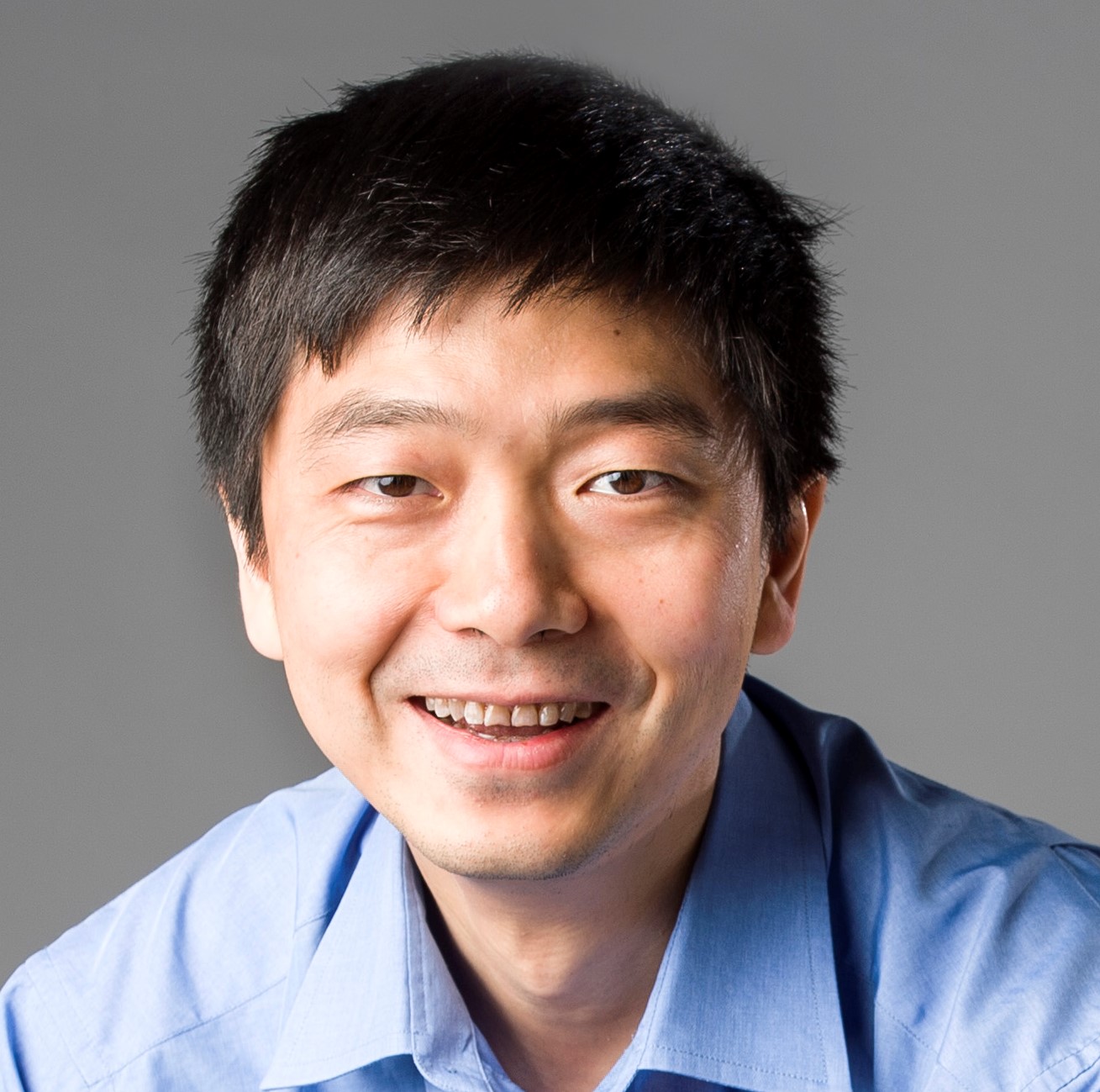
Hoifung Poon
Senior Director of Biomedical NLP at Microsoft
Hoifung Poon is the Senior Director of Biomedical NLP at Microsoft Research and an affiliated professor at the University of Washington Medical School.
He leads Project Hanover, with the overarching goal of structuring medical data for precision medicine. He has given tutorials on this topic at top conferences such as the Association for Computational Linguistics (ACL) and the Association for the Advancement of Artificial Intelligence (AAAI).
His research spans a wide range of problems in machine learning and natural language processing (NLP), and his prior work has been recognized with Best Paper Awards from premier venues such as the North American Chapter of the Association for Computational Linguistics (NAACL), Empirical Methods in Natural Language Processing (EMNLP), and Uncertainty in AI (UAI).
He received his Ph.D. in Computer Science and Engineering from the University of Washington, specializing in machine learning and NLP.