Measuring the Benefits of Healthcare Specific Large Language Models
There is overwhelming evidence from academic research and industry benchmarks that domain-specific and task-specific large language models outperform general-purpose LLMs across multiple dimensions: Accuracy, veracity, human preference, and cost.
This session presents the results of a double-blind study, in which medical doctors compared John Snow Labs’ healthcare-specific LLMs with OpenAI’s GPT-4o across four popular medical language understanding tasks:
- Medical text summarization, across a variety of patient notes and report types
- Open-ended medical question answering, testing out-of-the-box general medical knowledge
- losed-ended question answering – extracting specific information from a given patient note, such as a patient’s primary diagnosis or disease stage
- Closed-ended biomedical research – understanding a given research paper abstract
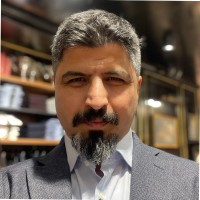
Veysel Kocaman
Head of Data Science at John Snow Labs
Veysel is a Head of Data Science at John Snow Labs, improving the Spark NLP for the Healthcare library and delivering hands-on projects in Healthcare and Life Science. Holding a PhD degree in ML, Dr. Kocaman has authored more than 25 papers in peer reviewed journals and conferences in the last few years, focusing on solving real world problems in healthcare with NLP.
He is a seasoned data scientist with a strong background in every aspect of data science including machine learning, artificial intelligence, and big data with over ten years of experience. Veysel has broad consulting experience in Statistics, Data Science, Software Architecture, DevOps, Machine Learning, and AI to several start-ups, boot camps, and companies around the globe.
He also speaks at Data Science & AI events, conferences and workshops, and has delivered more than a hundred talks at international as well as national conferences and meetups.