NLP for scaling healthcare access to everyone
Telemedicine is a rapidly growing medium of interaction with the healthcare system. The current pandemic has led to a 100% increase in virtual urgent care visits and a greater than 4000% increase in virtual non-urgent care visits.
Despite substantial growth in telemedicine, equitable access to the best healthcare continues to be a dream that is yet to be realized. Text-based telehealth services are poised to play an important role: it is affordable and accessible at the moment, and can, efficiently, scale operationally. The earliest research work at the intersection of AI/NLP and healthcare can be traced back to the 1960s, for instance with Eliza AI program for medical conversations, albeit with little practical success.
In recent years, there have been tremendous advancements in AI/NLP (e.g. BERT, GPT-3). What does this mean to healthcare?
In this talk, we explore two integral components to carry forward these advancements in NLP to healthcare: The first component is around encoding medical knowledge. While Eliza was medically accurate, it didn’t scale. Large scale machine learning models such as GPT-3 can easily scale but they currently lack the fidelity in high stake application of healthcare.
In this talk, we will present some of our research in harnessing these models to incorporate medical knowledge by incorporating data from diverse sources such as medical texts and EHRs. Second, is the issue of data availability. Access to large amounts of labeled data is hard to obtain – in addition to privacy concerns, medical data exhibits long-tail distribution for a good reason, e.g. some diseases are more common than others, some medical conversations are more common than others.
To overcome these challenges, we discuss our approach using active learning and designing large-scale models to be effective data labelers.
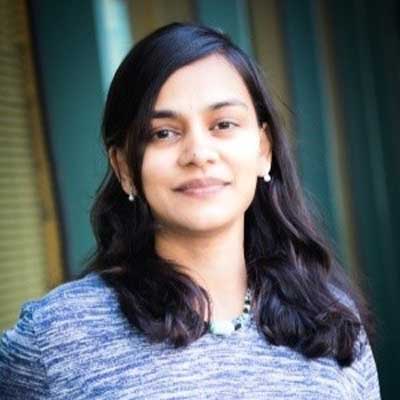
Anitha Kannan
Head of Machine Learning Research at Curai
Anitha Kannan is a founding member and Head of Machine Learning Research at Curai where she leads research on AI-driven innovative solutions to equitable best quality healthcare accessible to all.
Prior to Curai, she has held senior research positions at Facebook AI research and at Microsoft research.
She holds a Ph.D. in machine learning from the University of Toronto and was a Darwin Fellow at the University of Cambridge, UK.
She has extensively published in top-tier conferences and holds numerous patents.