No-Code Transfer Learning from Rules & Models in the Annotation Lab
The high-scale analysis of text and image data in verticals from Healthcare to Finance and Security relies more and more on the use of AI models and pipelines as part of broader intelligent applications. Up until now, AI models have been accessible to a handful of people: hands-on experts in deep learning and transfer learning. Our goal at John Snow Labs is to make them accessible to everyone!
This talk introduces Annotation Lab 4.0, a Free, End-to-End, No-Code platform that can be used by domain experts – nurses, doctors, lawyers, accountants, investors, etc. – to train high-accuracy NLP models from scratch or tune existing models to better respond to their use cases. Our tool supports the end-to-end process from starting an annotation project to the deployment of a trained model, all without writing a line of code. Based on an auto-scaling architecture powered by Kubernetes, it can scale to many teams and projects. Enterprise-grade security is provided for free including support for air-gap environments, zero data sharing, role-based access, full audit trails, MFA, and identity provider integrations. It allows powerful experiments for model training and finetuning, model testing, and model deployment as API endpoints.
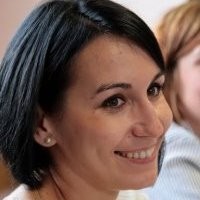
Dia Trambitas
Head of Product John Snow Labs
Dia Trambitas is a computer scientist with a rich background in Natural Language Processing. She leads the development of the Annotation Lab, currently the best-in-class tool for text and image annotation for healthcare.
Dia holds a Ph.D. in Computer Science focused on Semantic Web and ontology-based reasoning. She has a vivid interest in text processing and data extraction from unstructured documents, a subject she has been working on for the last decade. She has broad experience delivering information extraction and data science projects across Finance, Investment Banking, Life Science, and Healthcare.