Patient Similarity through Representation Learning from Medical Records
The assessment of patient similarity is a fundamental component of many secondary uses of medical data. In order to calculate distance, heterogeneous EMRs must be converted into comparable formats. We discuss a novel method of representing data in EMRs that considers information in clinical narratives.
Unsupervised method for building a patient representation is proposed, which integrates unstructured and structured data from patients’ electronic medical records. An EMR tree structure is used to model the extracted data that captures the temporal relationships between multiple medical events. In order to capture the temporal aspects of the events extracted from the tree, two new relabeling methods are presented.
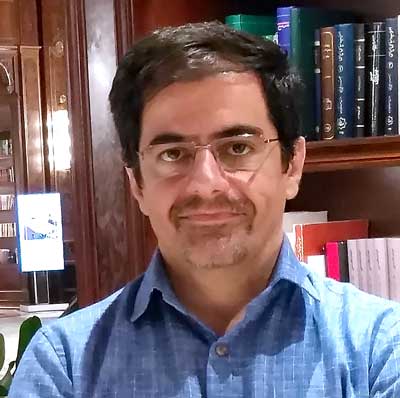
Nasser Ghadiri
Associate Professor Isfahan at University of Technology
Nasser is an Associate Professor at Isfahan University of Technology, where he develops models and NLP algorithms for extracting information from unstructured healthcare data. Moreover, he collaborated with bioinformaticians on drug repositioning algorithms that integrate data from heterogeneous sources to discover new applications for existing drugs. Currently, Nasser is a research fellow at Western Sydney University.