Sparse Expert Models: Past and Future
In this talk we will discuss the recent rise in popularity of sparse expert models (e.g. mixture of experts) and their implications for the NLP field. Spasity allows for networks to have inputs to get different subsets of the model weights, which allows for vastly larger models that have smaller computational footprints.
These networks have recently achieved state-of-the-art results on many famous NLP benchmarks while showing tremendous computational savings. We discuss the recent research advances and the future of what these models achieve and why it has large implications for the way machine learning models are used.
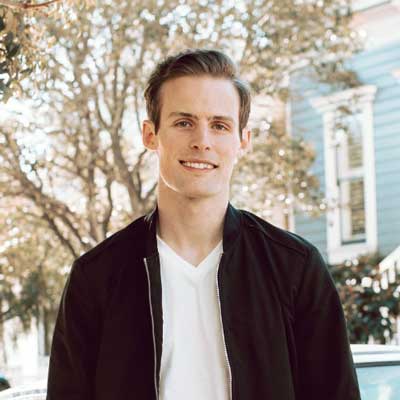
Barret Zoph
Research Scientist at Google Brain
Barret Zoph is a staff research scientist on the Google Brain team. He has worked on a variety of topics including Neural Architecture Search, semi-supervised learning, automatic data augmentation methods like AutoAugment and neural network sparsity such as Switch Transformer.
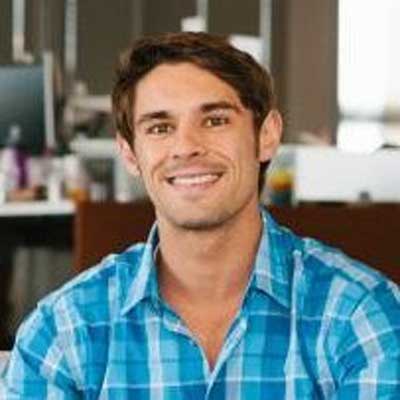
Liam Fedus
Member of the Technical Staff at OpenAI
Liam is a researcher focused on efficient and reliable large-scale machine learning systems. Previously, Liam was a Senior Research Scientist at Google Brain and is now a member of the Technical Staff at OpenAI.