The State Of The Art On Knowledge Graph Construction From Text
Knowledge graphs (KG) play a crucial role in many modern applications. Industrial knowledge is scattered in large volumes of both structured and unstructured data sources and bringing them to a unified knowledge graph can bring a lot of value. However, automatically constructing a KG from natural language text is challenging due the ambiguity and impreciseness of the natural languages. Recently, many approaches have been proposed to transform natural language text to triples to construct KGs.
This presentation aims to summarize the research progress over the KG construction from text with a specific focus on the information acquisition branch entailing entity and relation extraction covering the state of the art methods and tools. In this talk, we will provide an overview of the field of knowledge graph construction from text including the state of the art methods, techniques and tools for constructing knowledge graphs from text, their capabilities, limitations and current challenges. This will be useful for any practitioner who is interested in building knowledge graphs for their organizations.
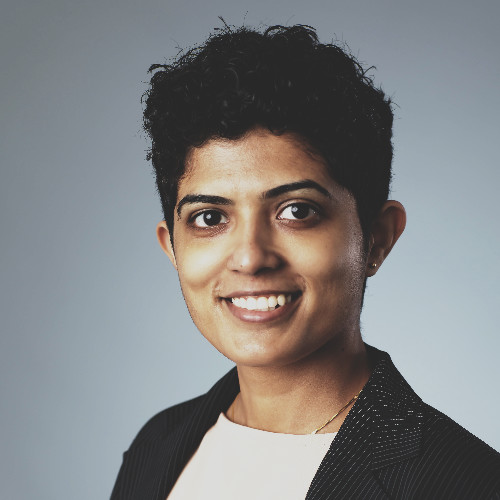
Jennifer D’Souza
Postdoctoral researcher at TIB – Leibniz Information Centre for Science and Technology
Jennifer works as a postdoctoral researcher on the Open Research Knowledge Graph project in the Data Science and Digital Libraries group at TIB. Her work experience is in developing unsupervised or supervised machine learning techniques for natural language processing (NLP). Her current research focus is on semantifying knowledge from scholarly text by NLP methods. Jennifer holds a PhD from the University of Texas at Dallas where her research focus was on relation mining from natural language text with a specific focus on time and space relations.
She have since worked as a postdoctoral researcher at the University of California, Davis, where she studied the application of natural language processing techniques to software engineering tasks under the umbrella of the Naturalness of Software initiative. Following this and before joining TIB, she spent a brief period in a startup environment in industry developing concept discovery software solutions.
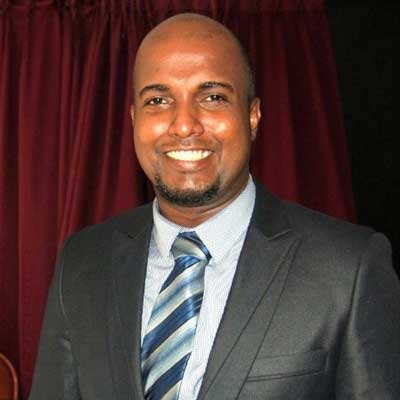
Nandana Mihindukulasooriya
Research Scientist at IBM
Nandana Mihindukulasooriya is a Research Scientist at MIT-IBM Watson AI Lab. His research interests include relation extraction and linking, information extraction, knowledge representation and reasoning, and Neuro-Symbolic AI.
Nandana holds a PhD in AI and has published more than 60 peer-reviewed papers in prestigious journals, conferences on Semantic Web and Knowledge Graph related topics.