TLDR: Extreme Summarization of Scientific Documents
Scientists are often required to process long lists of papers, such as conference proceedings and search engine results, and information overload is becoming an increasing problem for scientists. Titles often don’t convey enough information about the content of a paper, and abstracts are on average over 150 words in length, which can be time-consuming to read in large numbers.
We introduce TLDR generation or the automatic generation of extreme summaries for scientific literature. TLDRs are single-sentence summaries that communicate the main points of a paper and are commonly used on social media and websites like OpenReview.net.
In this talk, I will discuss the challenges of automatically generating TLDRs, including data collection, modeling, evaluation, and implementation, and how we addressed them.
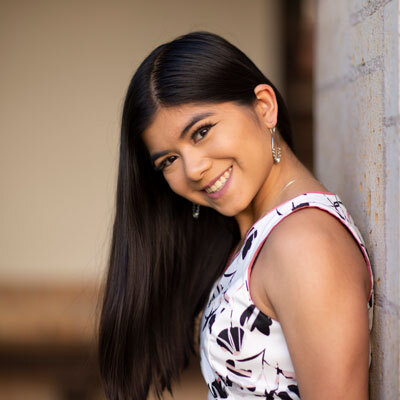
Isabel Cachola
Ph.D. Student at Allen AI / John Hopkins
Isabel Cachola is a second-year Ph.D. student studying Computer Science at Johns Hopkins, advised by Dr. Mark Dredze. Previously she was a Pre-Doctoral Researcher at the Allen Institute for AI on the Semantic Scholar team, advised by Dr. Dan Weld.
She earned her B.S. in Mathematics at the University of Texas at Austin, advised by Dr. Jessy Li and Dr. Greg Durrett. Her research interests include text generation, summarization, question answering, and computational social science.