What’s so hard about training and deploying LLMs?
In this talk, Davis Blalock will walk through the hurdles of memory limitations, hardware failures, compute management, experimentation, evaluation, and how they all contribute to the escalating time and cost of training LLMs. He will also discuss the reliable and efficient systems his team has built to address these challenges – enabling better, cheaper, faster workflows for organizations of all sizes to train and deploy generative AI models.
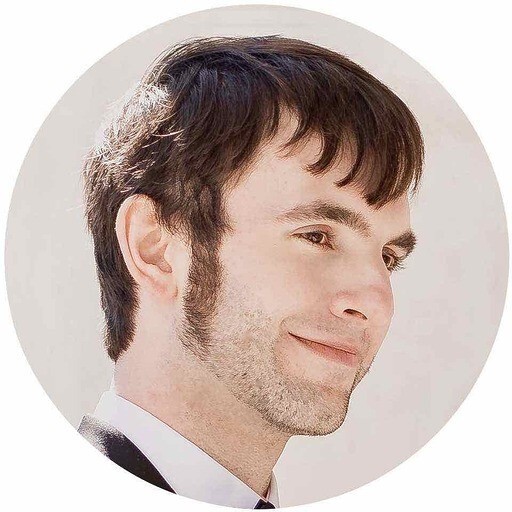
Davis Blalock
Research Scientist at MosaicML by Databricks
Davis Blalock, Research Scientist, Databricks
As the first employee of generative AI startup MosaicML, now acquired by Databricks, Davis is uniquely suited to breaking down the challenges of LLM training and deployment—and the tips and tricks he uses to make machine learning at scale accessible.
Davis also runs Davis Summarizes Papers, a blog read by 13k+ ML researchers and practitioners, where he goes through all the machine learning arXiv submissions each week and summarizes 10 to 20 of his favorites papers. Prior to MosaicML, Davis received his PhD from MIT, where he designed high-performance machine learning algorithms with the goal of eliminating tradeoffs between speed, accuracy, privacy, and safety in machine learning.